Orange, Calif. –Existing data on building destruction in conflict zones currently rely on eyewitness reports or manual detection, which makes it generally scarce, incomplete, and potentially biased. With satellite imagery becoming more ubiquitous, research has demonstrated that artificial intelligence applied to satellite imagery holds promise for automated detection of war-related building destruction. While these results are promising, monitoring in real-world applications requires high precision, especially when destruction is sparse and detecting destroyed buildings is equivalent to looking for a needle in a haystack.
In a new article, Jonathan Hersh, assistant professor of economics and management at Chapman University, Andrea Matranga, assistant professor of economics at Chapman University’s Smith Institute for Political Economy and Philosophy, and their colleagues introduce an automated method of measuring destruction in high-resolution satellite images using deep-learning techniques combined with label augmentation and spatial and temporal smoothing.
The team processed the images through an artificial intelligence (CNN) model. Then, they processed the artificial intelligence’s predictions through a second machine learning stage. This second stage looked at temporal and spatial anomalies that would lead the artificial intelligence to update its probability that a building is damaged.
Published in Proceedings of the National Academy of Sciences of the United States of America (PNAS), this research demonstrates that exploiting the persistent nature of building destruction can substantially improve the training of automated destruction monitoring. As a proof of concept, the researchers applied their automated method to the Syrian civil war and reconstructed the evolution of damage in major cities across the country.
“We believe this research shows that, using the artificial intelligence outlined in the article, it is feasible to create an automated, real-time detector of bombing damage that shows where violence is occurring anywhere in the world,” said Hersh, Ph.D., who teaches at the Argyros School of Business and Economics at Chapman. “There are still problems to be solved before this can be operationalized, but we believe this is a huge leap forward.”
This approach allows generating destruction data with unprecedented scope, resolution, and frequency—and makes use of the ever-higher frequency at which satellite imagery becomes available.
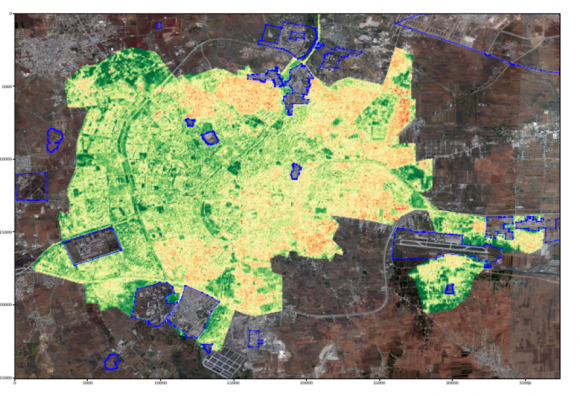
The paper also makes a significant contribution in using machine learning for situations where there are limited examples of the event occurring. By using reasonable contextual information about the event, the researchers showed that they can greatly increase the number of usable examples.
“We observed buildings in Aleppo over 23 different time periods, but only know with certainty whether a building is damaged or not for four of those time periods. However, using the reasonable assumption that no reconstruction occurs over the time period, we greatly increased the number of examples. More examples allow our artificial intelligence model to be much more precise than if it only saw a smaller number of examples,” said Hersh.
The researchers seek to expand on the methods pioneered in this study by scaling their algorithm to other locations where violence might be occurring and by building a website to present an early warning indicator of bombing damage for humanitarian use.
The research paper is titled “Monitoring war destruction from space using machine learning.” Hersh and Matranga were joined in the research by Hannes Mueller at the Institute for Economic Analysis (IAE), Spanish Council for Scientific Research (CSIC); and Andre Groeger and Joan Serrat at The Autonomous University of Barcelona.
###
About Chapman University
Founded in 1861, Chapman University is a nationally-ranked private university located in Southern California. Chapman is categorized by the Carnegie Classification as an R2 “high research activity” institution and offers personalized education to more than 9,000 undergraduate and graduate students. The campus has produced a Rhodes Scholar, been named a top producer of Fulbright Scholars and hosts a chapter of Phi Beta Kappa, the nation’s oldest and most prestigious honor society. Based in the City of Orange, Chapman also includes the Harry and Diane Rinker Health Science Campus in Irvine. In 2019, the university opened its 11th college, Fowler School of Engineering, in its newest facility, Keck Center for Science and Engineering. Learn more about Chapman University: www.chapman.edu